Epistemic vigilance in the age of AI
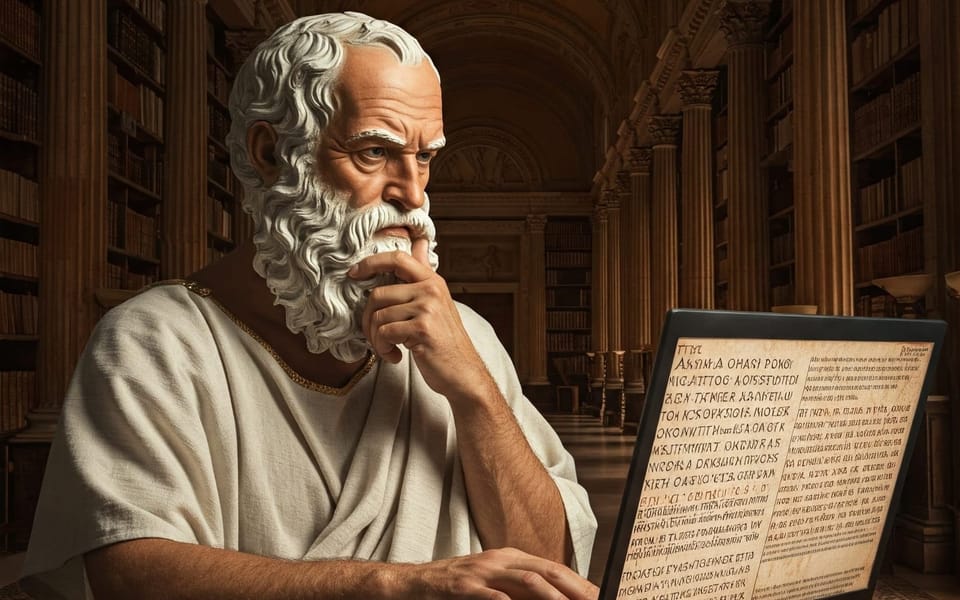
When ChatGPT was introduced to the world at large, the first concerns in education were about plagiarism. Students could use the technology to generate essays, research proposals, reading summaries, and much more, which meant many common assessment forms were suddenly less valid – they were no longer reliable indices of the knowledge and skills of students. Or at least, they would not be reliable indices if students would choose to use LLMs for their work.
The second wave of concerns what about whether any of that mattered. Was writing an essay still a valuable skill? Why were we asking students to write those in the first place? What would we be losing if we just let students integrate LLMs in into their knowledge work? These questions became all the more pressing as more and more professionals did exactly that, leading to hypocritical situations in which teachers were banning tools from their classes, while at the same time using them to do their research or even to create their classes.
One phrase that keeps popping up in these conversations is training critical thinking. If knowledge tasks are performed too smoothly, critics say, there is no opportunity to think critically about the content in the same way that truly writing a paper by yourself does. If students use LLMs for knowledge generation, advocates say, they do need to remain vigilant and should maintain a critical attitude, so that their use of the technology is responsible.
In the next series of posts, I want to explore how we might be able to teach students to think critically about LLM output. I also want to see whether LLMs could be used by students to improve critical thinking skills. In both cases I want to depart from the notion that humans are natural-born arguers and that we are "epistemically vigilant" – we tend to not believe just anything we hear or read. Still, I think there are some unique obstacles in this particular case:
- The cognitive machinery underlying our epistemic vigilance was optimised for human-human interactions. It might not work well in this new technological environment.
- LLMs were built to generate linguistic forms, not knowledge in the sense of justified, true beliefs. If two humans try to figure something out, critical thinking will emerge, but in human-LLM interactions only one of the two is trying to figure something out.
But there are reasons for optimism, too. To the extent that dialogue prompts us to think more deeply, LLMs should be able to take on this role. To the extent that our critical thinking is limited by the options we can see, the astronomical association capacity of LLMs might open our minds. At the university where I work, pilots using generative AI in education suggest that students take on a critical stance towards LLM output quite naturally.
A first step when designing education for this purpose is to bring some order into the chaos. As I've often mentioned on this blog, critical thinking is a container concept, covering a wide range of cognitive skills and dispositions. Unfortunately, LLM use is equally varied, with people trying to throw it against any sort of cognitive task they can think of. Looking at these huge sources of variation and tying some knots between them will the topic of the next post.
Member discussion